Emerging artificial intelligence (AI) technologies are starting to play an increasingly dominant role in business today. As a subset of AI, Machine Learning (ML) uses massive amounts of data and algorithms to “imitate” the way humans learn without being programmed to perform specific tasks or functions. It powers numerous applications, including chatbots and predictive text suggestions, your favorite streaming app as it learns what you like to watch, and healthcare apps that diagnose medical conditions based on images.
Companies from retail to manufacturing, banking to legacy enterprises use ML to unlock new value or boost operational efficiency. While ML is complex and may seem hard to grasp, business and industry leaders must at least understand its basic principles, potential, and limitations, as it’s here to stay.
In this article, we’ll cover the current trends in AI, and in particular machine learning (ML), how ML is being used to optimize business processes and operations, and what lies ahead for it and the business world.
Current Trends in Machine Learning and Artificial Intelligence
More industries are now leveraging AI and ML to meet evolving customer needs and accelerate digital transformation. The expansion of edge computing and Internet of Things (IoT) devices, generate more data for training ML models that power operational excellence.
Here are some of the top trends in AI and ML right now:
1. Companies are adopting open data ecosystems.
Since data is the key to unlocking ML, accelerating operational efficiency, and increasing return on investment (ROI), companies are moving to an open data ecosystem, where all data is stored in a single database or data warehouse, where it can be analyzed and mined for trends holistically. This eliminates data silos, simplifies data integration, and facilitates multi-cloud analytics.
2. Machine Learning infuses insights across the organization.
Companies that revise their analytics strategies to incorporate ML improve decision-making, speed up new product development, and increase customer acquisition and retention by providing every team with the information they need on demand.
3. Machine Learning sheds light on unknown data.
ML can help companies discover, classify, and manage structured and unstructured data more efficiently. This allows you to better understand your data and draw insights from analysis of that data.
4. Machine Learning powers the next generation of cybersecurity.
AI and ML will play a growing role in detecting and responding to tomorrow’s cybersecurity threats. Companies can use ML’s learning capabilities to defensively and proactively detect anomalous behavior and identify network vulnerabilities. For example, credit card companies are using it to help detect fraudulent behavior.
How Artificial Intelligence Drives Operational Improvement
ML is a powerful tool for driving automation across an organization and can handle more complex processes that standard, rule-based automation cannot. It’s ideally suited for this complexity, as it is trained using updated information on an on-going basis, such that over time it becomes more accurate and efficient.
Some companies use ML in their manufacturing processes as part of their inspection phases to speed up inspection timelines, reduce errors, and make corrective changes upstream earlier than they could before. For example, biopharmaceutical company Amgen uses ML to maximize the efficiency of their visual inspection systems and cut false syringe rejects by 60%.
Retailers and other inventory-driven companies can use ML models to monitor product levels in real time and make changes according to local factors. Levi Strauss, the jean manufacturer, uses ML models to predict the demand for their products globally and rolls out adaptive pricing automatically as needed.
Today’s companies must learn how to move beyond the individual ML use case to organization-wide adoption, something many need help with. Learning how to clearly explain the benefits and impacts of ML on the organization is one way to break through the barriers. Another way is by starting small and optimizing your company’s repetitive processes.
The Role of Repetitive Process Optimization
Some processes are vital to a company but take time and effort. Optimizing these repetitive processes with ML models is an ideal entry point. ML can analyze, suggest, learn, and adapt based on the data generated by the processes.
Take this large U.S.-based retailer that used ML to better understand and forecast the cash requirements for their stores. Instead of manually surveying the stores or having store managers report in their reserves, they used an ML tool to analyze historical data and build a predictive 30-day cash requirement for each store. Because the tool was more than 97% accurate, it helped reduce capital requirements and saved the retailer more than $4 million annually.
Other repetitive processes ideal for ML optimization include data entry and validation, invoice processing, payment validation, customer support, sales lead scoring, and marketing campaign identification.
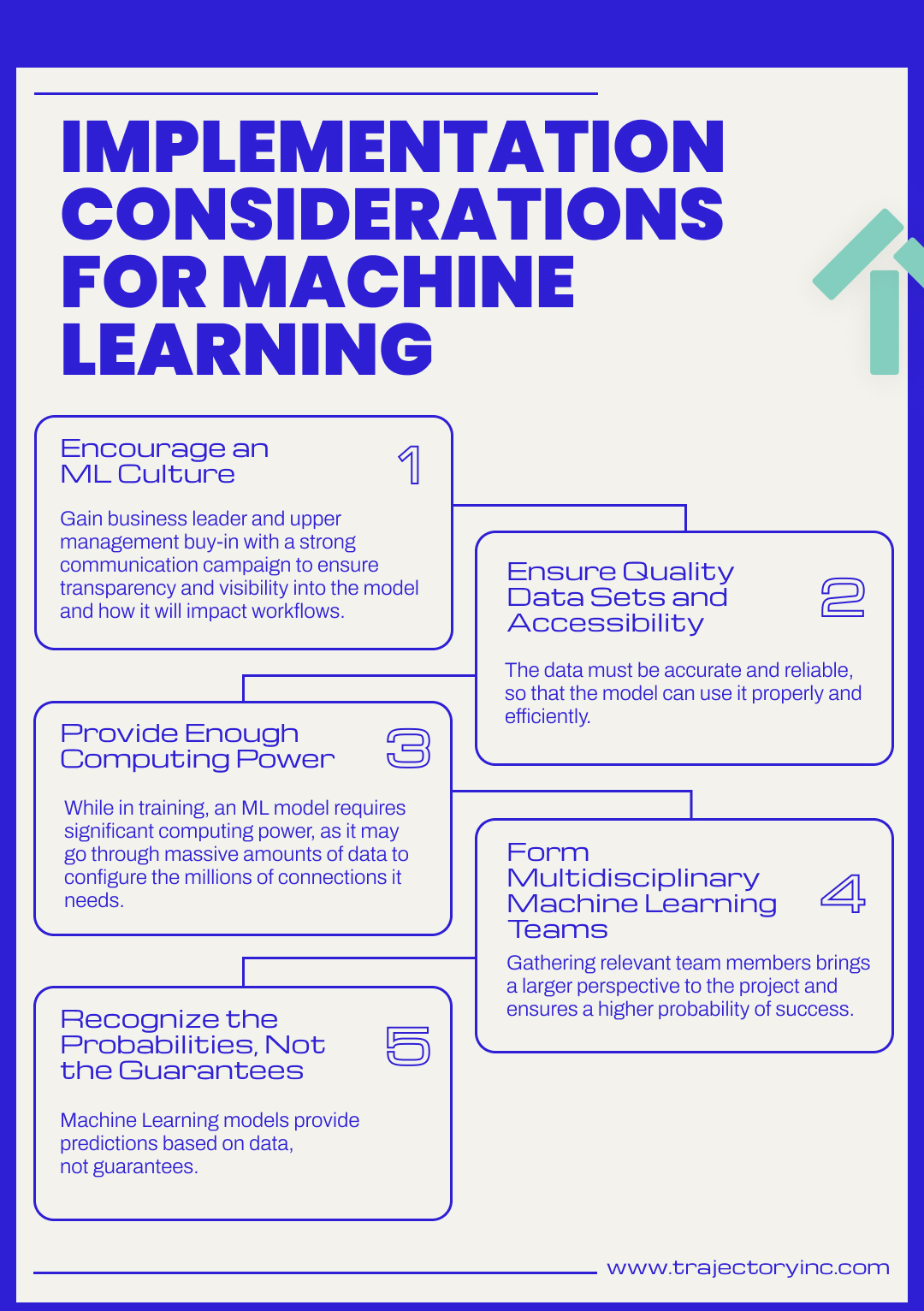
Operationalization Considerations for Machine Learning
Machine learning models can help from the start, but they improve with time and access to real-world data. Putting an ML model into production environments where they’re accessing and analyzing real-world data and offering actual predictions is called “operationalizing” the ML model rather than implementing it.
That’s because the models can be applied to many different problems and situations, and they reside everywhere, from a desktop computer to an edge computing device or a cloud server environment. Each place where the model operates is considered a separate deployment.
To ensure a successful operationalization of your ML model, here are a few keys to success:
1. Encourage an ML Culture
The biggest change from adding ML to your business is the cultural change that must accompany operationalization. Gain business leader and upper management buy-in with a strong communication campaign to ensure transparency and visibility into the model and how it will impact workflows.
2. Ensure Quality Data Sets and Accessibility
ML models are only as good as the data they have access to and analyze. As the adage goes, garbage in/garbage out, so your model needs access to relevant and clean data. The data must be accurate and reliable so the model can be used it properly and efficiently.
3. Provide Enough Computing Power
While in training, an ML model requires significant computing power, as it may go through massive amounts of data to configure the millions of connections it needs. Depending on the required training, some businesses may need higher-powered graphics processing units (GPUs) to start. However, once completed, ML models occupy significantly less space so you can dial back the computing power at that point.
4. Form Multidisciplinary ML Teams
While an ML model is fundamentally made up of technology that performs a function, the data and business processes make it relevant and work properly. Gathering a multidisciplinary team of IT team members, data scientists, and relevant business team members brings a larger perspective to the project and ensures a higher probability of success.
5. Recognize the Probabilities, Not the Guarantees, of ML
ML models provide predictions based on data, not guarantees. When operationalizing ML, include processes for when it doesn’t provide the results with acceptable levels of certainty. That means adding thresholds for when a result is acceptable and alternate functionality if it falls outside those thresholds. Otherwise, investing in ML may be a mistake if you don’t have the right expectations upfront.
The Future of Operational Improvement with Artificial Intelligence
As AI and ML become more sophisticated, they will impact the business world in broader ways. Companies will use models to solve more complex classification, prediction, and control problems as ML moves into spoken audio, video, and image realms. It could be used to anticipate people’s needs, deliver solutions proactively, create customized user experiences, identify gaps in business processes, and ensure compliance with regulatory and legal guidelines.
McKinsey predicts that soon, ML will use natural language processing (NLP) to handle live customer support calls. It could potentially understand a customer’s mindset, emotional state, and the nature of their concern in live calls, reducing the headcount needed to staff certain business functions and freeing them up to work on more value-adding projects.
Other future uses of ML models include:
- Combining inputs from sensors to operate autonomous vehicles.
- Developing computer-vision-enhanced safety procedures that increase employee safety across high-risk industries like construction and manufacturing.
- Using ML for pathology recognition and diagnosis-decision support in healthcare to enhance patient diagnosis and care.
Preparing for this ML-powered future means increasing funding to relevant teams, finding the right talent to guide ML during its lifecycle, addressing cybersecurity concerns, and understanding how ethical, regulation, and compliance issues may affect AI and ML research and applications.
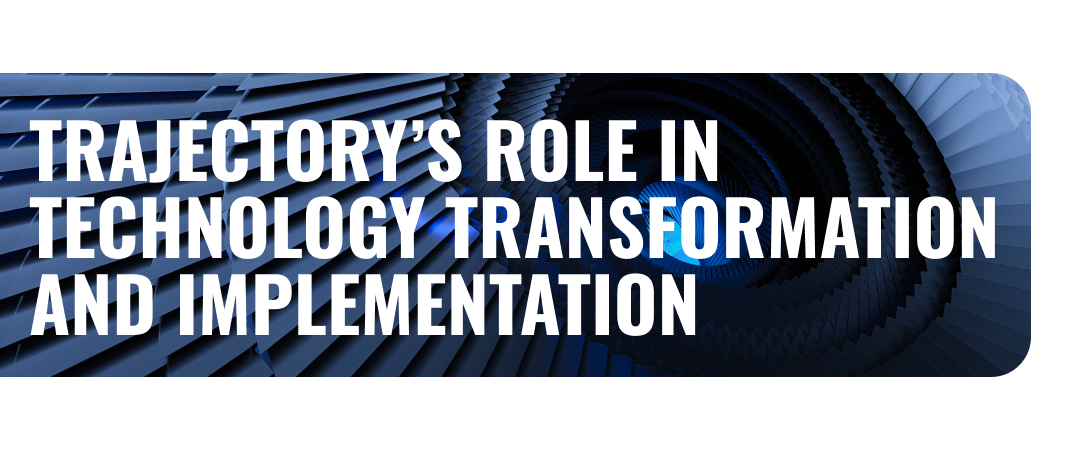
In the words of Alex Olano Trajectory’s Chief Executive Officer and Managing Partner, “Operational improvement has become a buzzword with little business impact.” That’s because many companies add new technologies thinking they alone will make the difference when it rarely does. Successfully transforming a company involves the right balance of technology, process improvements, and a shift in employee perspective.
As experts in digital transformation, Trajectory team members follow a business-first, solution-agnostic approach. We’re focused on the “what” and “how” of any optimization project. We work with key stakeholders to understand our clients’ businesses, refine their processes, and create a clear roadmap that outlines the full solution and ecosystem to achieve their business goals.
Trajectory client Corsearch Inc., a trademark clearance and protection company, appreciated this holistic approach in their work with us. “Trajectory started with an assessment of business processes and systems. They didn’t treat it as a templated solution. They carefully reviewed our setup and business processes and identified realistic solutions,” Satoru Ogawa, Chief Financial Officer of Corsearch, said.
This approach is especially important when implementing machine learning models—it’s a complex process that some business leaders think is the solution to everything. Artificial Intelligence is a technology solution that supports business process refinement and drives the company toward its ultimate objectives when implemented well.
ML is the latest tool in the business leader’s toolkit starting to pay dividends. Companies use it to identify new opportunities, fix gaps in business processes, and streamline overall business functions to be more efficient. Machine learning can be used across the organization, whether to create new marketing campaigns that improve customer engagement, predict when equipment needs repair or replacement, or optimize inventory and staffing levels.
To take control of your company’s path to success and drive it forward with machine learning, contact Trajectory today. We are ready to help you start your AI journey.
About the Author – Vlad Olano – VP Operations, Managing Partner